Core Topics
The morphology of membranes determines their properties and thus their potential in sustainable components and separation processes. Consequently, a detailed characterization of the structure creates the basis for the development of new nanostructured polymers and membranes. For this purpose, our department performs morphological as well as chemical analysis using imaging techniques. Electron microscopic, atomic force microscopic and light microscopic methods are employed for this purpose.
To interpret the image data, a holistic view of the membrane, including the details of polymer synthesis and membrane production, is essential. This coupling of data from different departments and their management is conducted in electronic laboratory notebooks. The accumulation of these large, structured and complex data sets can be used for evaluation with statistical and machine learning methods that far exceed the intuition of the individual scientist.
-
Morphology -
Chemical composition - Electronic laboratory notebook and data management
-
Machine Learning Data Analytics

Scanning electron micrograph of a block copolymer membrane, cross section and surface.
Photo: Hereon
Light microscopes, electron microscopes (SEM, TEM) and an atomic force microscope (AFM) are used for the investigation of the membrane strucuture, also under conditions close to the application in liquids and with multidimensional modes such as TEM tomography and QNM-AFM. These multidimensional images represent a virtualization of reality, which then allows validation of the digital twins. Besides, they are used to evaluate the membrane fabrication and support all other departments.
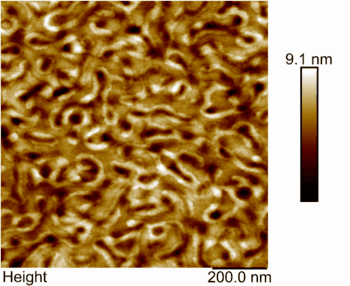
Multimodal imaging of a block copolymer using AFM.
Different modes highlight different aspects of phase separation.
Figure: Hereon
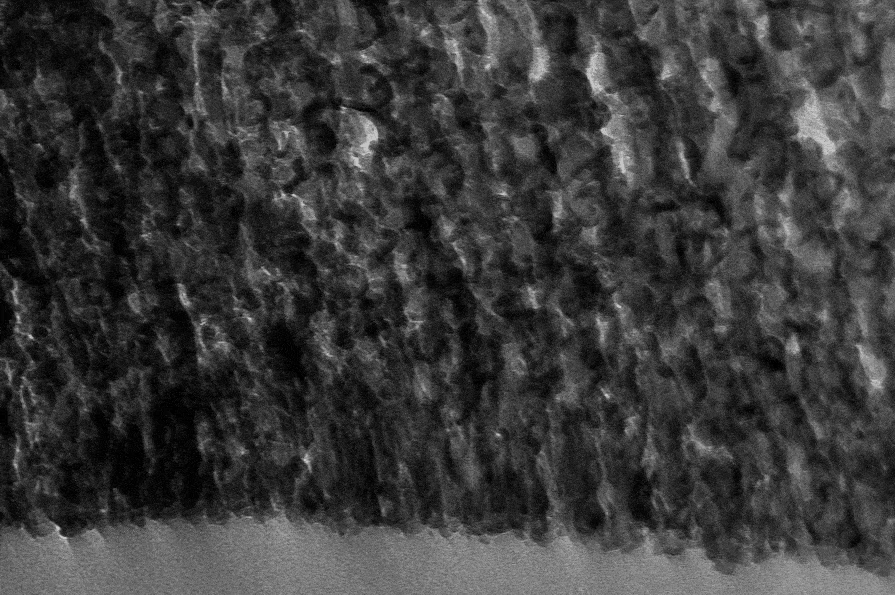
TEM tomography for 3D imaging of a block copolymer membrane. Contrast enhancement of the pores by aluminum oxide.
Figure: Hereon
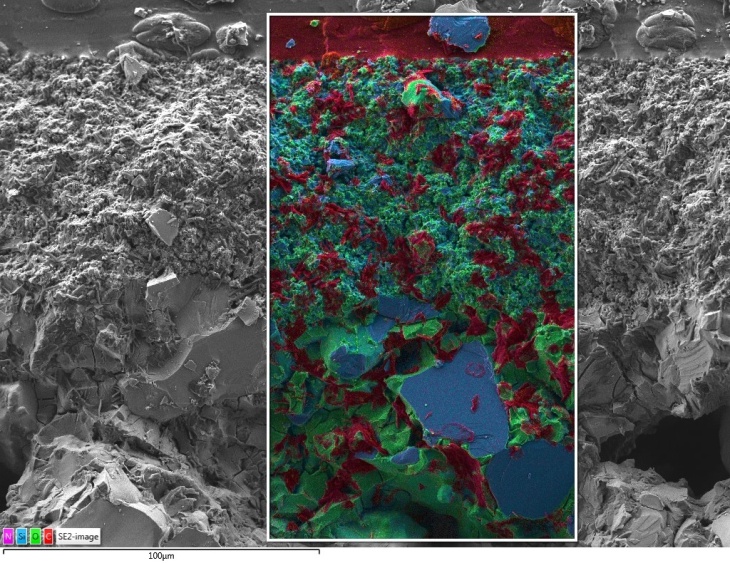
Chemical identification by means of characteristic X-rays (EDX). The coloration of the chemical elements allows the interpretation of the pure morphology.
Photo: Hereon
Mere imaging of the morphology often does not allow an assignment of the individual chemical components of a membrane or of filler materials. Spatially resolved investigations of the chemical composition to identify the individual phases are performed using selective contrasting, energy dispersive X-ray spectroscopy (EDX), energy-filtered transmission electron microscopy (EFTEM), electron energy loss spectroscopy (EELS) and Raman microscopy. These methods complement the microscopic images and often make the full interpretation of the imaging techniques possible.

Chemical identification by selective contrasting of different polymer blocks with 2 contrast agents.
From: DOI 10.1002/adma.201907014
Photo: Hereon
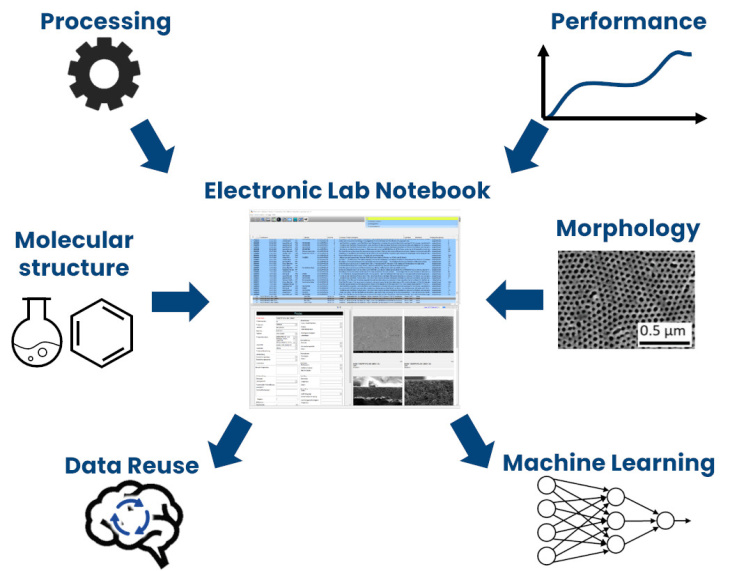
Figure: Hereon/Martin Held
In essence, the imaging techniques represent a digitization of the morphology and composition of real membranes. The resulting image data are stored in an image database by data management. This is coupled with two electronic laboratory notebooks (ELNs), and thus with the parameters of synthesis, membrane preparation and permeation properties. Our data thus become FAIR: on one hand, the ELNs allow the data to be retrievable and accessible for decades. Second, by linking them to other data via an identification number, the data remain interpretable because the context of synthesis and analysis is preserved. All of this promotes the reusability of our data treasure, especially through data scientific methods such as machine learning.
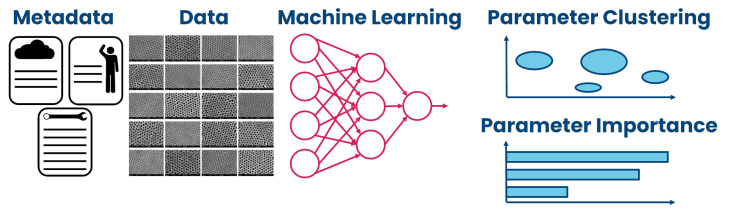
Figure: Hereon/Martin Held
The optimization of membranes and the discovery of new membrane parameters takes place in a high-dimensional parameter space. Experimental approaches cannot test the entirety of this space, although part of it has already been explored and documented using data management. By analyzing the data coupled through the electronic lab books using machine learning and artificial intelligence methods, the understanding of the process-structure-property relationships will be extended and prediction of future membrane properties will be enabled. For example, image data will be analyzed in the context of synthesis and manufacturing and performance data to highlight the relevance of process parameters. For this, the creation of suitable image descriptors is essential.