Amanda Barnard visits the Institute of Biomaterial Research
Amanda Barnard works as a chief scientist at Data61, a research division of the Australian national science research agency, CSIRO. Data61 grapples with the wide ranging topic of digitalisation. Barnard heads the area for material science and brings new computational methods to the research, such as self-learning algorithms.
![The interview was recorded at the Institute for Biomaterial Research in Teltow. [Photo: HZG/Gesine Born] Amanda Barnard and journalist Frank Grotelüschen.](/imperia/md/images/hzg/presse/pressemitteilungen/2019/fittosize__730_0_52db42df19953a7a5afb6ecda18ffe2f_interview_amanda_barnard-2.jpg)
The interview was recorded at the Institute for Biomaterial Research in Teltow. [Photo: HZG/Gesine Born]
The 47 year-old physicist is seen as a pioneer in her field and has been recognised with many awards, including the renowned “Feynman Prize in Nanotechnology” from the Foresight Institute. In September, she visited the Institute of Biomaterial Science in Teltow to speak to the researchers there about her work and future co-operations.
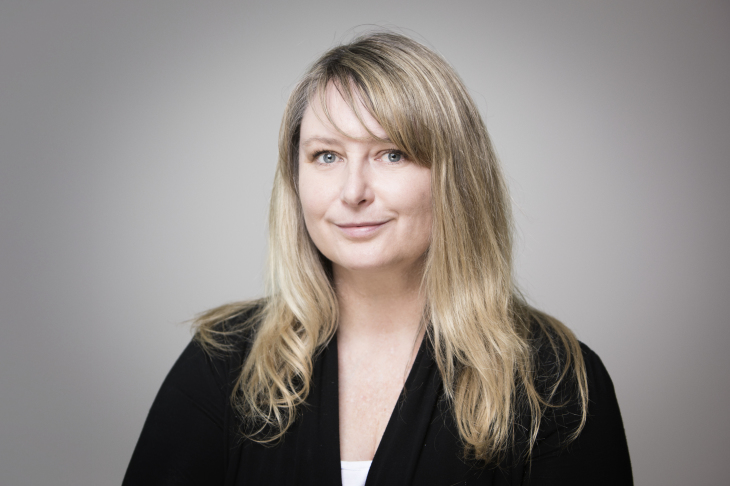
Amanda Barnard ist mit zahlreichen Preisen ausgezeichnet worden, darunter dem renommierten „Feynman Prize in Nanotechnology“ des Foresight Instituts. [Foto: HZG/Gesine Born]
Material science seeks to develop new materials with improved properties and functions -for example, materials that are lighter, longer-lasting and more efficient. Computational models and simulations have long played an important role.
What has happened up till now, and how do researchers make these simulations more powerful?
Amanda Barnard: Traditionally the methods used in computational material science have had a deterministic approach: starting with a theory, implementing them on a supercomputer and generating predictions that can be used in very specific situations. If we wanted to be able to do more predictions, we need to go back to the start and gather more information and start again every time. Using a data-driven approach, we can make multiple predictions by applying different kinds of computational algorithms to the same dataset, providing insights that would be hidden if we used any other approach.
Therefore, the starting point for these new approaches is data. Are data from experiments the most important, or can data from other sources be used?
Amanda Barnard: One of the advantages that we have in our part of Data61 is that we have a background in the theories of materials, physics, and chemistry, and we're able to generate our own synthetic datasets, which is a huge advantage. This kind of degree of control is something that experimentalists don't currently have. But we also like to work with experimental datasets, because they include all of the realism and complexity of the real world.
What are the challenges? What do you need to pay attention to in order to ensure that a self-learning algorithm, for example, actually reveals something new and useful in the data?
Amanda Barnard: Eighty percent of the time is spent making sure that the data is right. It's rather tedious, but it's absolutely necessary. Machine learning is very sensitive to things like biases, variations in the feature space or the way you describe your data and any kind of missing data or problems that exist. Another challenge for a material scientist is that they don't have big data. They have little data. One of the challenges of our research is developing ways that the same methods can be used on smaller datasets, and sparse datasets, and sets that have a lot of other faults in them, which the inventors of machine learning never anticipated.
![Künstliche Intelligenz und maschinekkes Lernen sind auch in der Wissenschaft Modebegriffe geworden. [Foto: Markus Spiske on Unsplash] Computercodes](/imperia/md/images/hzg/presse/pressemitteilungen/2019/fittosize__730_0_be8f3c4a3dfa6600802d3e019578bfc3_photo_by_markus_spiske_on_unsplash.jpg)
Künstliche Intelligenz und maschinekkes Lernen sind auch in der Wissenschaft Modebegriffe geworden. [Foto: Markus Spiske on Unsplash]
How can machine learning or artificial intelligence be used in material science? Could you give me an example?
Amanda Barnard: One of our projects in collaboration with the University of New South Wales is to predict the catalytic activity of bi-metallic nanoparticles. This combines experimental results from our collaborator who makes nanoparticles and then characterises them. We then generate computational synthetic data samples on high-performance supercomputers and then do data fusion to combine these two data sets together and have a greater resource to predict what kind of structures will be most catalytically efficient. Catalytic nanoparticles are generally made from very expensive rare metals. And if we can reduce the amount of very expensive platinum or palladium, for example, we can drive the costs down.
Terms like artificial intelligence and machine learning have become buzzwords. However, for many they continue to sound mystical or even terrifying.
Amanda Barnard: I don't find them mystical or terrifying at all. I find them quite exciting. Artificial intelligence simply performs a task for us. It's not really replacing scientific knowledge so much as informing scientific experts on the best course of action. It doesn't replace any other methods we already have, but provides us a new opportunity, a new tool in the toolkit that we haven't had before.
And what do your fellow researchers think about these new opportunities? Are they open or are they sceptical?
Amanda Barnard: I think there's still a lot of sceptics. Certainly it's a very popular topic at conferences. But I'm not sure everyone's sold on this as a new way of doing research just yet. I think we're headed in the right direction. I think early career researchers have a very strong part to play. They are a lot more receptive to new ideas, and provided they can convince their professors that it's also a good idea, I think we'll get greater adoption.
![Das Interview mit Amanda Barnard führte der Journalist Frank Grotelüschen. [Foto: HZG/ Gesine Born] Amanda Barnard and journalist Frank Grotelüschen.](/imperia/md/images/hzg/presse/pressemitteilungen/2019/fittosize__730_0_6fc37d4d12ff6db39fba79b2a7ec7985_interview_amanda_barnard-5715.jpg)
Das Interview mit Amanda Barnard führte der Journalist Frank Grotelüschen. [Foto: HZG/ Gesine Born]
What roles do you predict the methods of artificial intelligence will play in ten years? What do you hope might happen?
Amanda Barnard: Actually I hope I can't predict, because it would be a very boring ten years if I already knew now what was going to happen! I hope I'm surprised many times over the next ten years. But I do expect that data-driven design of materials will become commonplace in laboratories all around the world. The greatest advantage will be that we can circumvent taking the wrong path. Hopefully, using data-driven design prior to laboratory experiments will give hints that can enable us to do the right experiments earlier in our research. We can save on resources, and we can save on our time.
Until now you’ve mostly been working in nanotechnology. Would you like to work on other themes, perhaps together with the Institute of Biomaterial Science in Teltow?
Amanda Barnard: Yes, we are very interested to get more experience in biomaterials, and I look forward to the opportunity to collaborate with the Helmholtz Zentrum Geesthacht. This is certainly something that we're already talking about.
Thank you so much for the interview.
Contact
Helmholtz-Zentrum Geesthacht
Centre for Materials and Coastal Research
- Institute of Biomaterial Science -
Kantstr. 55
14513 Teltow
Germany